Statistical Methods in Criminology: An In-Depth Analysis of Data-Driven Crime Studies
Introduction
Criminology, the scientific study of crime, criminal behavior, and the criminal justice system, has evolved significantly over the decades. A pivotal component in this evolution has been the integration of statistical methods. As societies become increasingly data-driven, the importance of using statistical tools to understand, interpret, and predict criminal behavior becomes more critical than ever. This article explores the profound role that statistical methods play in criminology, providing a comprehensive overview of methodologies, applications, and future directions. It highlights how statistical thinking transforms raw data into meaningful insights, allowing criminologists to address crime more effectively.
The Role of Statistics in Criminology
Statistics in criminology serve several core purposes that collectively enhance the understanding of crime and the efficiency of law enforcement and judicial processes:
Descriptive Analysis:
These are used to summarize the basic features of crime data. Descriptive statistics help identify crime patterns, such as which types of crimes are most common, where and when they occur, and who the victims and offenders are. This type of analysis serves as a foundation for more complex statistical explorations.
Inferential Statistics:
Inferential techniques allow criminologists to draw conclusions about larger populations based on data collected from smaller, representative samples. This approach is vital for studying populations that are difficult to measure directly, such as unreported crimes or marginalized communities.
Predictive Modeling:
These models aim to forecast future crime trends or potential offenders. By using historical data and identifying risk factors, criminologists and law enforcement agencies can develop strategies to prevent crimes before they occur.
Policy Evaluation:
Statistical methods are indispensable for evaluating the effectiveness of criminal justice policies and interventions. By comparing pre- and post-intervention data, analysts can determine if a program, such as a new policing strategy or rehabilitation initiative, is achieving its intended outcomes.
The integration of these statistical tools provides criminologists with a robust framework for making informed decisions and proposing actionable solutions.
Historical Background of Statistical Methods in Criminology
The history of statistical methods in criminology is deeply rooted in the broader history of social science. In the 19th century, Belgian astronomer and statistician Adolphe Quetelet was among the first to apply statistical methods to social phenomena. He introduced the concept of the “average man” and emphasized that human behavior, including criminal actions, could be studied through statistical regularities. His work laid the groundwork for empirical criminology.
Cesare Lombroso, often considered the father of modern criminology, also employed early forms of data analysis to support his biological theories of criminal behavior. Although many of his ideas have been discredited, his emphasis on empirical observation set a precedent for future research.
As the 20th century unfolded, the discipline expanded to incorporate sociological and psychological dimensions, moving away from purely biological explanations. The development of computers and statistical software in the latter half of the century revolutionized the field, enabling more sophisticated analyses and the handling of larger datasets.
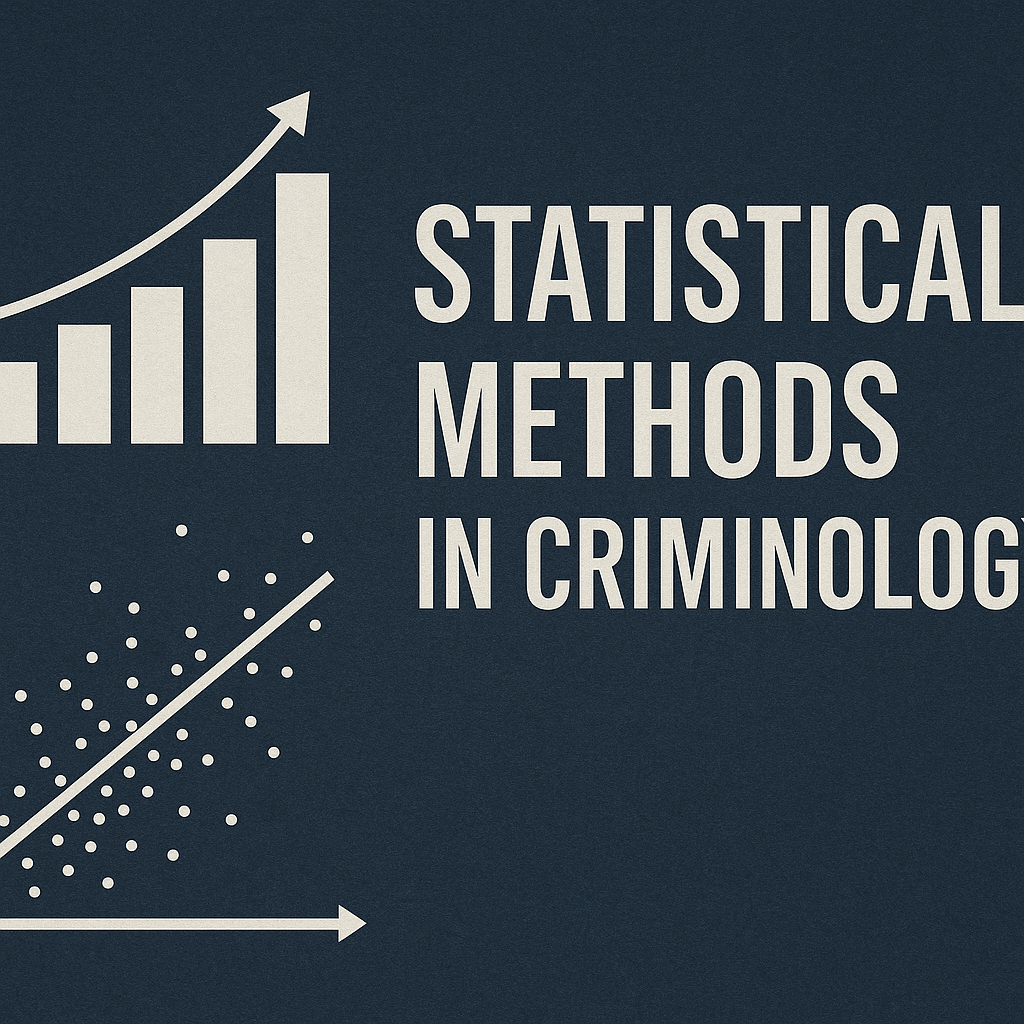
Types of Statistical Methods Used in Criminology
1. Descriptive Statistics
Descriptive statistics are foundational tools in criminology. They provide an overview of crime data and enable comparisons across different groups and time periods. Examples include:
- Measures of central tendency: These help identify the “typical” value in a dataset. For instance, the mean age of offenders or the median income level in high-crime neighborhoods.
- Measures of dispersion: These indicate the variability in the data. A high standard deviation in arrest rates across cities might suggest inconsistencies in enforcement practices.
- Frequency distributions: Often used to show how frequently certain types of crimes occur within a given period or location.
These methods are often used in official crime reports and by law enforcement agencies to communicate trends and inform resource allocation.
2. Inferential Statistics
Inferential statistics allow researchers to generalize findings from a sample to a broader population. They are essential when direct measurement is impractical or impossible. Common methods include:
- Hypothesis testing: Used to determine whether observed differences (e.g., crime rates between neighborhoods) are statistically significant.
- T-tests and ANOVA: Employed to compare means between groups, such as comparing recidivism rates between those who underwent rehabilitation programs and those who did not.
- Chi-square tests: Useful for analyzing categorical data, such as the relationship between gender and types of crime committed.
- Regression analysis: Helps identify relationships between independent variables (e.g., unemployment, education level) and dependent variables (e.g., crime rate).
3. Multivariate Analysis
Multivariate techniques are used when researchers need to analyze more than two variables simultaneously. They are especially useful in complex social phenomena like crime, where multiple factors interact. Examples include:
- Multiple regression: Allows the inclusion of several predictors in a single model.
- Factor analysis: Identifies underlying variables, or factors, that explain patterns in the data.
- Cluster analysis: Groups cases or variables that share similar characteristics, often used in profiling offenders.
- Structural Equation Modeling (SEM): Combines factor analysis and regression for modeling complex relationships among variables.
4. Time Series Analysis
Time series analysis helps researchers understand how crime rates evolve over time. This is crucial for detecting seasonal variations and long-term trends. Methods include:
- Autoregressive models (ARIMA): Predict future values based on past data.
- Seasonal decomposition: Separates time series data into trend, seasonal, and irregular components.
- Forecasting techniques: Used by law enforcement to plan for expected changes in crime rates.
5. Spatial Statistics
Geographic patterns of crime are analyzed using spatial statistics, which are crucial for urban criminology and environmental design. These include:
- Hotspot mapping: Identifies areas with high concentrations of crime.
- Geographic Information Systems (GIS): Used to visualize and analyze spatial data.
- Spatial autocorrelation: Measures the degree to which similar crime rates are clustered in space.
These tools are widely used in urban planning and law enforcement to enhance crime prevention strategies.
6. Bayesian Statistics
Bayesian methods offer a probabilistic framework that allows researchers to update beliefs based on new evidence. Unlike traditional frequentist methods, Bayesian analysis incorporates prior knowledge, making it particularly valuable when dealing with limited or uncertain data. Applications include risk assessment, decision-making in uncertain environments, and updating predictions as more data becomes available.
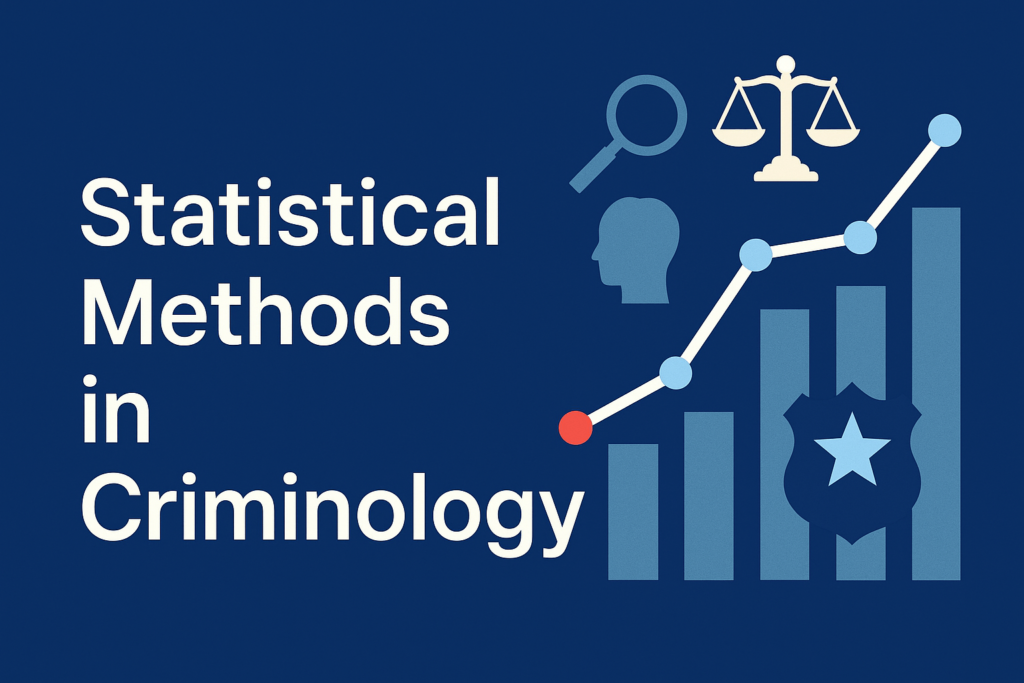
Applications of Statistical Methods in Criminology
Crime Mapping and Hotspot Analysis
Statistical tools are used to analyze spatial data and identify crime hotspots—specific locations where crimes occur with high frequency. Law enforcement agencies use this information to allocate resources more efficiently and design targeted patrol strategies. Hotspot analysis can also help in urban design by identifying poorly lit or isolated areas that may be more prone to criminal activity.
Predictive Policing
Predictive policing utilizes historical data and statistical algorithms to forecast where and when crimes are likely to occur. This proactive approach enables police departments to deploy resources more strategically, potentially preventing crime before it happens. However, it also raises ethical concerns regarding privacy, data bias, and the potential for reinforcing existing disparities.
Victimization Studies
Victimization surveys, such as the NCVS, provide insights into the prevalence of crimes that are not reported to law enforcement. Statistical analysis of this data helps identify vulnerable populations, understand the context of victimization, and evaluate the effectiveness of victim support programs. These studies are crucial for a comprehensive understanding of crime beyond official statistics.
Policy Impact Evaluation
Governments and organizations rely on statistical methods to evaluate criminal justice policies. For example, regression discontinuity designs and randomized controlled trials (RCTs) are used to assess the impact of interventions like body-worn cameras, community policing programs, or sentencing reforms. Such evaluations inform policy adjustments and ensure that public funds are used effectively.
Profiling and Risk Assessment
Statistical models are employed to assess the likelihood that an individual will reoffend (recidivism). These tools, often used by parole boards and judges, consider factors such as criminal history, age, employment status, and family support. While helpful, they must be used cautiously to avoid unjust discrimination.
Data Sources in Criminology
Reliable statistical analysis depends on high-quality, representative data. Key sources include:
- Uniform Crime Reports (UCR): A nationwide, annual summary of crime reported to police.
- National Incident-Based Reporting System (NIBRS): Provides detailed information on individual crime incidents.
- National Crime Victimization Survey (NCVS): Gathers data on both reported and unreported crimes through household surveys.
- Self-report surveys: Used in academic research to explore offending behavior that may not be captured by official data.
- Court and prison records: Offer insights into criminal processing and incarceration trends.
- Social media and open-source intelligence: Emerging sources for real-time data and public sentiment analysis.
Challenges in Applying Statistical Methods
Despite their power, statistical methods are not without limitations:
- Data Quality: Poor data quality—due to errors in reporting, underreporting, or inconsistent data collection—can compromise the validity of findings.
- Ethical Concerns: Collecting and analyzing crime data raises privacy and consent issues, particularly when involving vulnerable populations.
- Overreliance on Quantitative Methods: Numbers may fail to capture the lived experiences and motivations behind criminal behavior, underscoring the need for qualitative insights.
- Complexity and Accessibility: Advanced statistical techniques can be difficult for non-specialists to understand or apply, highlighting the importance of user-friendly tools and training.
The Importance of Statistical Literacy in Criminology
To fully leverage the power of data, criminologists and practitioners must be statistically literate. This involves understanding basic statistical concepts, being able to interpret research findings critically, and avoiding common pitfalls such as confusing correlation with causation. Statistical literacy enables professionals to:
- Design robust studies
- Interpret data accurately
- Communicate findings effectively
- Advocate for evidence-based policies
Educational programs in criminology should emphasize statistical training to prepare students for the demands of modern research and practice.
Future Directions in Statistical Criminology
Integration with Big Data
The explosion of data from digital sources—such as surveillance cameras, social media, and smartphones—offers new opportunities for crime analysis. Big data analytics enables the examination of patterns at unprecedented scales and speeds.
Machine Learning and AI
Artificial intelligence and machine learning algorithms can process large datasets to detect hidden patterns, classify crime types, and predict criminal behavior. These technologies hold promise but require careful oversight to avoid algorithmic bias and ensure fairness.
Interdisciplinary Approaches
Combining statistical methods with insights from psychology, sociology, and computer science leads to richer analyses and more effective interventions. Interdisciplinary collaboration is key to addressing the multifaceted nature of crime.
Open Data and Transparency
Promoting open access to crime data enhances transparency, fosters public trust, and encourages collaborative research. It also allows communities to hold institutions accountable and engage in data-driven advocacy.
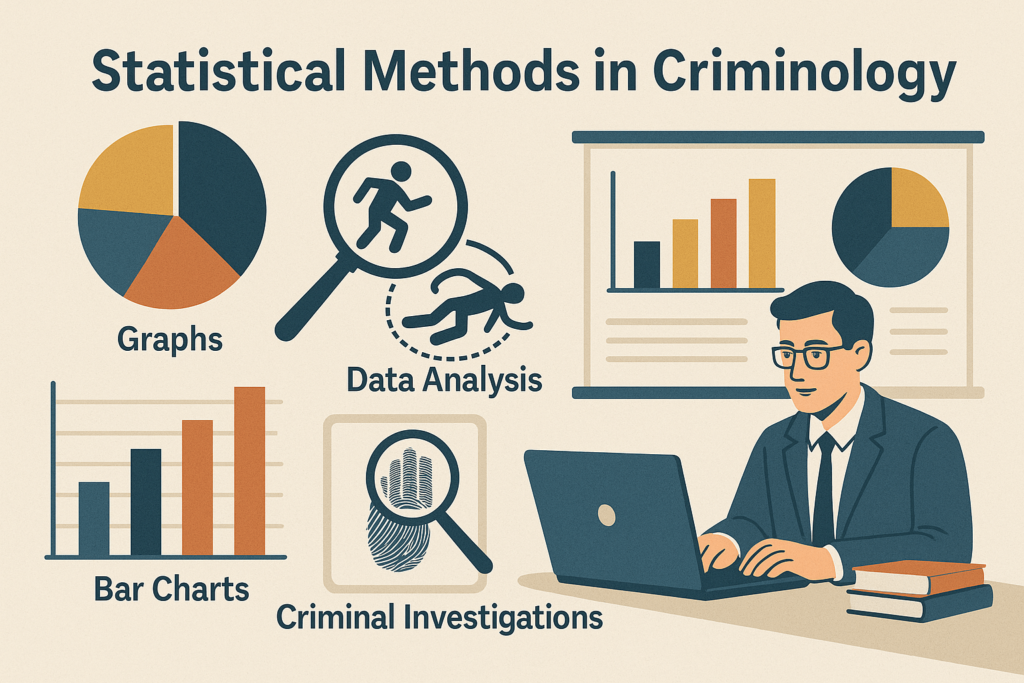
Conclusion
Statistical methods in criminology are indispensable tools for understanding the dynamics of crime and improving the justice system. From analyzing historical trends to forecasting future criminal behavior, these techniques enable evidence-based policy, ethical decision-making, and efficient resource allocation. As the field continues to evolve, embracing advanced statistical tools and maintaining rigorous standards of analysis will be critical for driving meaningful change in society. By fostering statistical literacy and encouraging interdisciplinary collaboration, criminology can continue to adapt to emerging challenges and remain at the forefront of social science research.